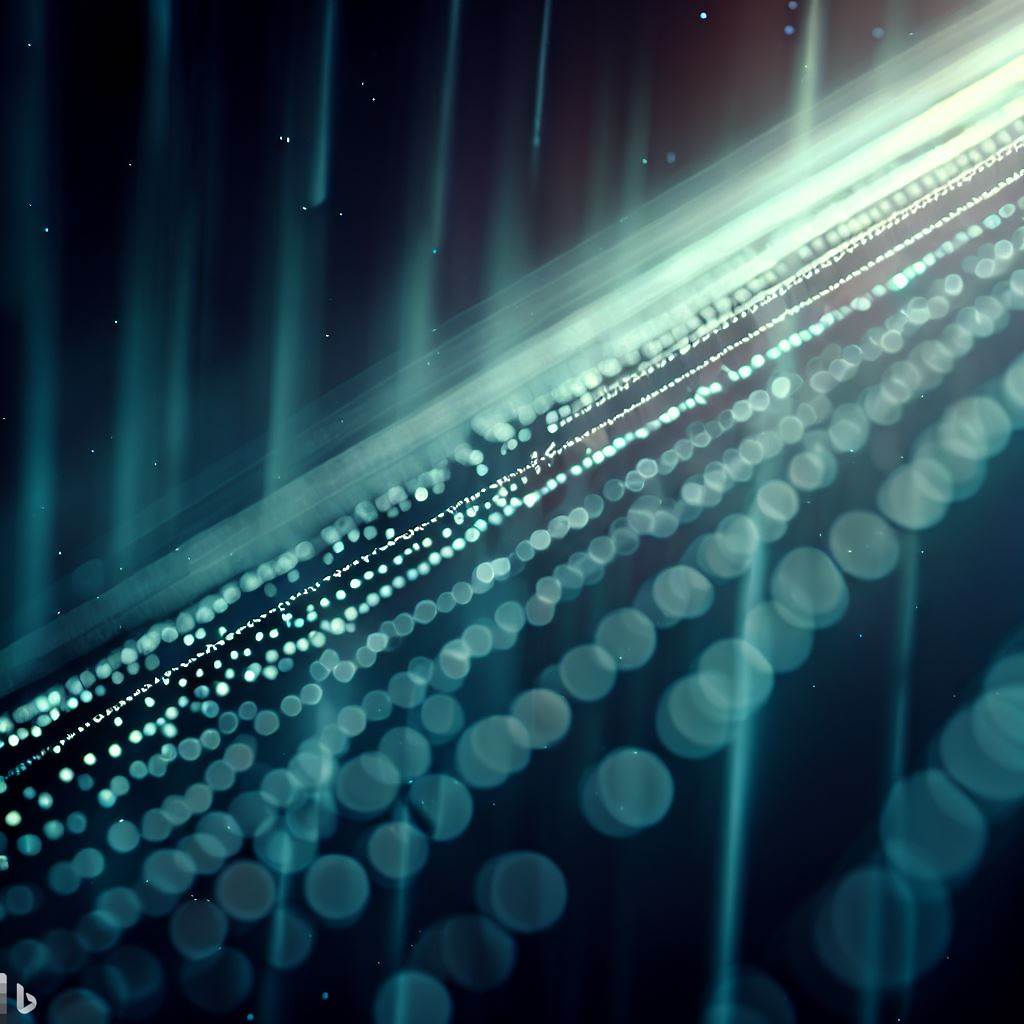
1๏ธโฃ You are predicting an average, not an actual value! When running a regression model, you’re finding the relationship between input variables and the mean value related to the outcome. It’s important to understand that the predicted value is an estimate of the mean of all possible values. Remember to communicate the uncertainty by using prediction intervals that capture the range of values.
2๏ธโฃ There is an expectation of a normal distribution for the outcome. The errors or residuals of your model should follow a bell curve. Check the distribution of residuals using histograms or QQ plots to assess how well your model fits the data. The closer the residuals align with a perfect normal distribution, the more confident you can be in your modeled mean.
3๏ธโฃ When your process is multiplicative, an important transformation is needed. In linear modeling, we add up multiple input variables assuming they have an additive effect. However, when dealing with probability or multiplicative processes, the outcome variable needs to be transformed to an additive process. Use logarithms to convert the multiplicative process into an additive one. In logistic regression, we model the log-odds and then exponentiate the coefficients to interpret odds ratios.
๐ Understanding these three key aspects of regression modeling will improve your approach to linear and generalized linear modeling. Remember, a model is only as good as your understanding of it, so dive deep into the math and trust the results with confidence! ๐๐ฌ
Leave a Reply